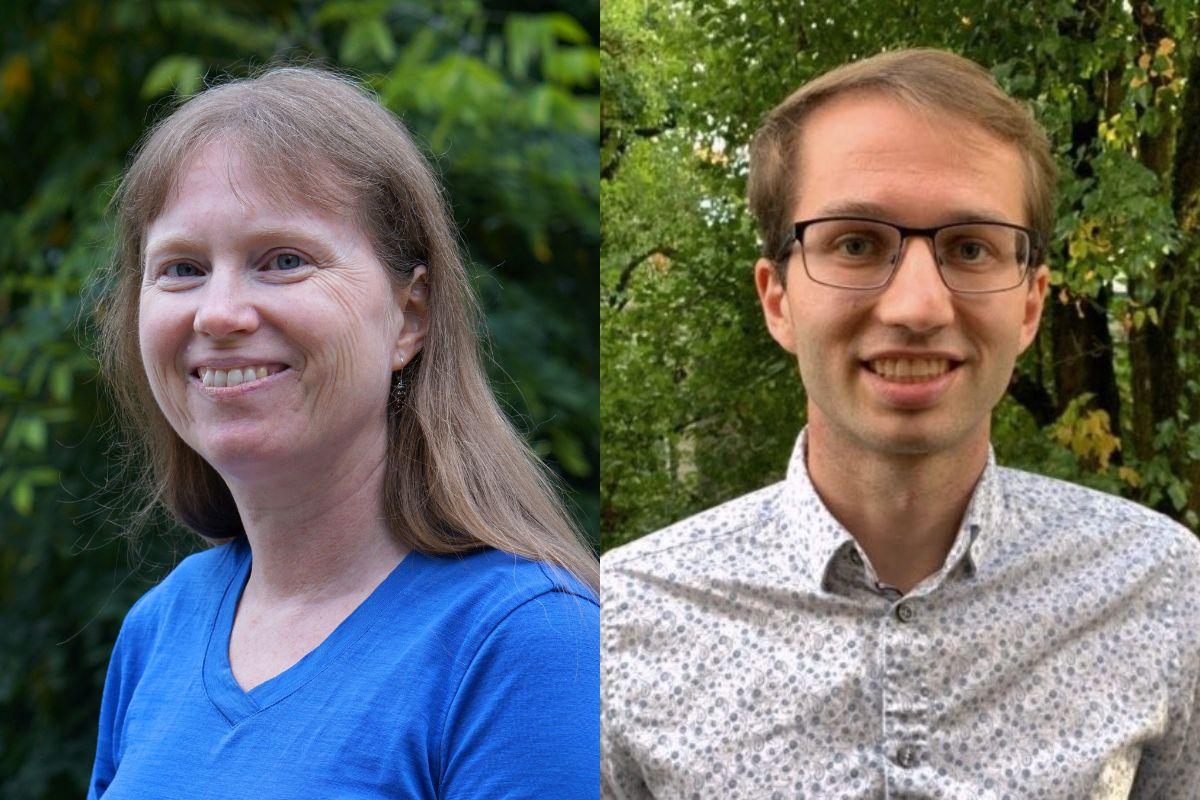
Most of us are familiar with the concept of positive selection, the evolutionary process where advantageous genetic variants increase rapidly in frequency, which allows populations to better adapt and survive. A classic example is selection at the lactase persistence gene that allows people with a specific variant to digest lactose in milk after childhood.
Two main challenges in modeling this process are determining if a genetic variant is under selection and measuring the speed at which its frequency changes. To address this, a team of University of Washington (UW) researchers recently proposed statistical methods that are more robust and accurate to study and understand the current and past frequencies of unknown adaptive alleles. The paper was recently published in The American Journal of Human Genetics.
"Our method detects signatures of strong natural selection that have occurred in humans in the past several thousand years," said Sharon Browning, senior author of the study and a professor in biostatistics.
“Many of the signals that we find are in genes that play a role in immunity, and likely represent responses to past pandemics as well as to common pathogens. These results can point to how the human genome has evolved to meet the challenges coming from new and evolving infectious diseases. Learning about these genetic changes could be useful in the design of new vaccines and treatments.”
First author Seth Temple, who recently graduated from UW with a PhD in statistics and now works as a postdoc at the University of Michigan, related some of the challenges the team encountered while developing the method.
“People may be familiar with testing hypotheses for statistical significance and reporting confidence intervals. We provide a rigorous framework to test if a genetic variant, among millions of genetic variants, is under selection while avoiding making too many false positive results. We also provide a method to make 95% confidence intervals for a selection strength parameter that contain its true value 95% of the time. To date, it has been difficult to achieve these properties when modeling selection with data only from the present day.
“We consider scientific claims about rapid adaptive evolution to be strong conclusions. They could be misinterpreted or misconstrued to say that some phenotypic traits are better than others. This concern is why developing methods that properly quantify uncertainty is important,” said Temple.
Data and Code Availability
The published article includes a Python package and automated bioinformatics pipelines written in snakemake. The iSWEEP software is freely available under the open source CC0 1.0 Universal License (https://github.com/sdtemple/isweep).