All humans are a genetic mix of different historical populations that have intermingled over time. This merging of gene pools results in newly formed groups referred to as admixed.
Analyzing genetic data from admixed individuals to infer local ancestry is valuable for genetic association analysis because it pinpoints genes involved in causing disease. But until recently, there weren’t any computational methods capable of managing the vast amounts of data required to conduct this analysis in a robust way.
This changed when a team of University of Washington researchers developed a method called FLARE (Fast Local Ancestry Estimation), which is outlined in a paper that appeared in the American Journal of Human Genetics.
“We developed FLARE because existing software for inferring local ancestry was not able to handle the increasingly large amounts of genetic data that are available,” said Sharon Browning, a professor of biostatistics and lead researcher of the team that also included Brian Browning, a professor of medical genetics and adjunct professor of biostatistics, and post-doctoral fellow Ryan Waples.
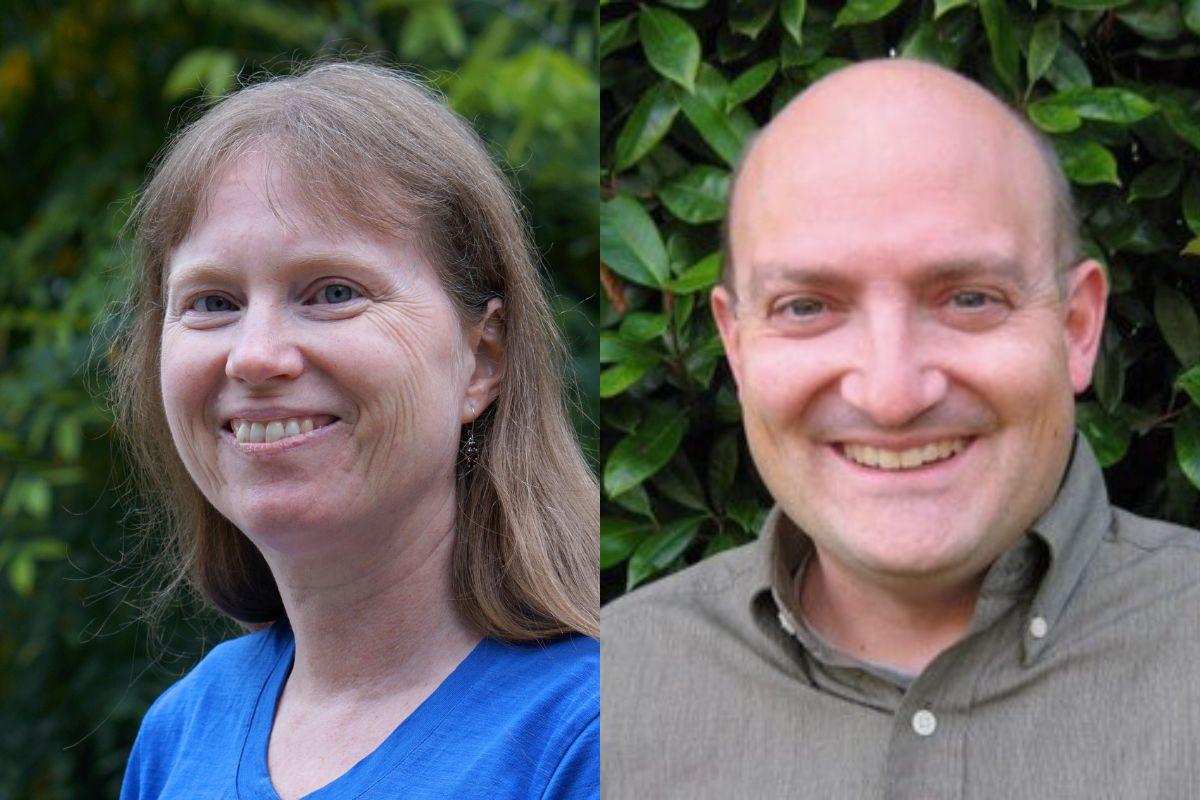
“Existing methods could handle hundreds of thousands of genetic variants across the genomes of thousands of individuals, but we wanted to apply local ancestry inference to whole genome sequence data with hundreds of millions of genetic variants on hundreds of thousands of individuals.”
Browning explained that local ancestry inference refers to taking genetic data from individuals who have ancestry from more than one part of the world and figuring out what part of those individuals' genomes are derived from each ancestry.
“’Local’ refers to figuring out the ancestry at each location in the genome, as opposed to ‘global’ ancestry’ which is the overall proportion of each ancestry in an individual.”
The team faced two major challenges in developing FLARE.
One was the computational challenge of making FLARE fast while not sacrificing accuracy, a problem solved by adapting techniques they had developed for other large-scale analyses of genetic data (haplotype phasing and genotype imputation).
The other complication was estimating parameters for FLARE's statistical model. FLARE uses genetic data from parent reference populations as its basis for inferring ancestry in admixed individuals.
“Some of FLARE's parameters reflect the relationships between the reference populations and the ancestries in the admixed individuals. Other parameters relate to admixture time and admixture proportions, and how a chromosome from one individual can be modeled as a mixture of chromosomes from other individuals. Each of these parameters is critical to the success of the local ancestry inference, and each required a different approach for estimation,” said Browning.
It was important to the team that FLARE be accessible to other scientists.
“We put a lot of emphasis on developing software that is robust and easy for other researchers to use. A lot of work went into developing the software and its documentation, and we also make an ongoing effort to provide support to researchers using the program,” said Browning.
Two research collaborations currently use FLARE. One is applying FLARE to the Hispanic Community Health Study genetic data, where it will be used for genetic association analysis for various diseases, including kidney disease. The other collaboration is applying FLARE to populations in the Africa6K project, where it will be used to study population histories.
The FLARE software is available from https://github.com/browning-lab/flare. The simulation and analysis pipeline used in this study is available from https://github.com/rwaples/lai-sim.